Stock assessment typically involves developing a set of alternative models, fitting each to the available data, and then selecting the one that gives the most accurate estimates of management quantities of interest. In this context, it is important to consider model selection uncertainty because ignoring it can lead to unreliable estimates and overconfident inferences. For this study, four Bayesian surplus production models with symmetric or asymmetric production functions and either a constant or hierarchical time varying intrinsic growth rate (r) were developed using data for Pacific blue marlin (Makaira nigricans) and Western and Central North Pacific striped marlin (Kajikia audax). The uncertainty resulting from model selection was evaluated using Monte Carlo simulation techniques to examine the consistency of model estimates within (self-tests) and among (cross-tests) the alternative models. Specifically, these tests evaluated the performance of the deviance information criterion (DIC) and Bayesian model averaging (BMA).
The results of the simulation tests suggested that mis-specification of time-varying r can lead to large estimation errors for biomass and management quantities and that DIC may not reliably identify the true data-generating model. Although BMA did not provide more accurate point estimates than just selecting the data-generating model, it did provide a more accurate characterization of uncertainty in model results.
Our study shows the value of using simulations to evaluate model performance and to account for model selection uncertainty.
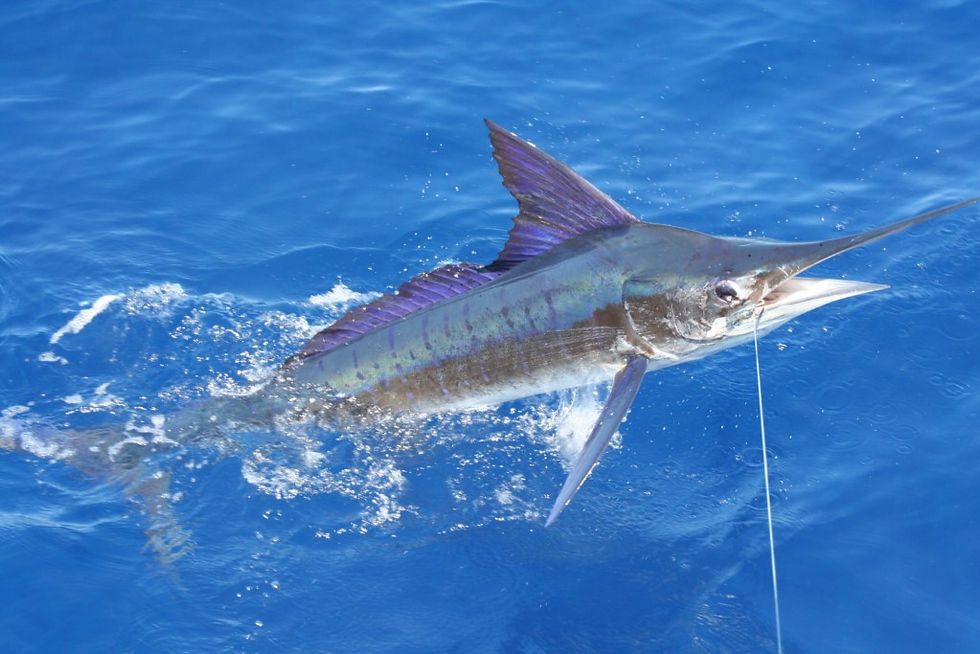
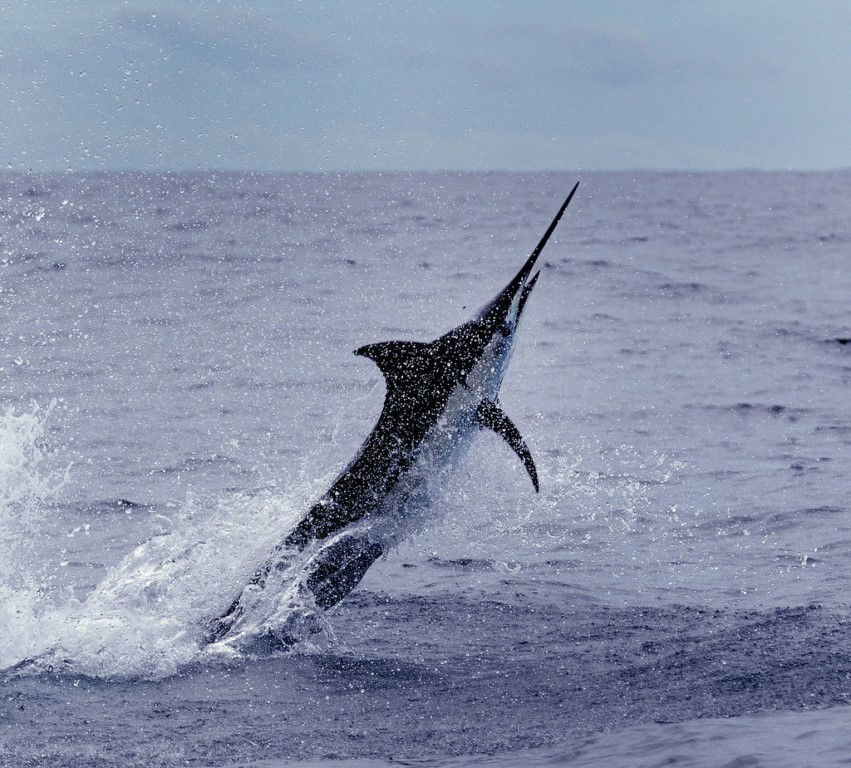
Source: Chang Y.J., Brodziak, J., O’Malley, J., Lee, H.H., DiNardo, G., and Sun, C.L. (2015) Model selection and multi-model inference for Bayesian surplus production models: A case study for Pacific blue and striped marlin. Fisheries Research, 166: 129-139.
Comments